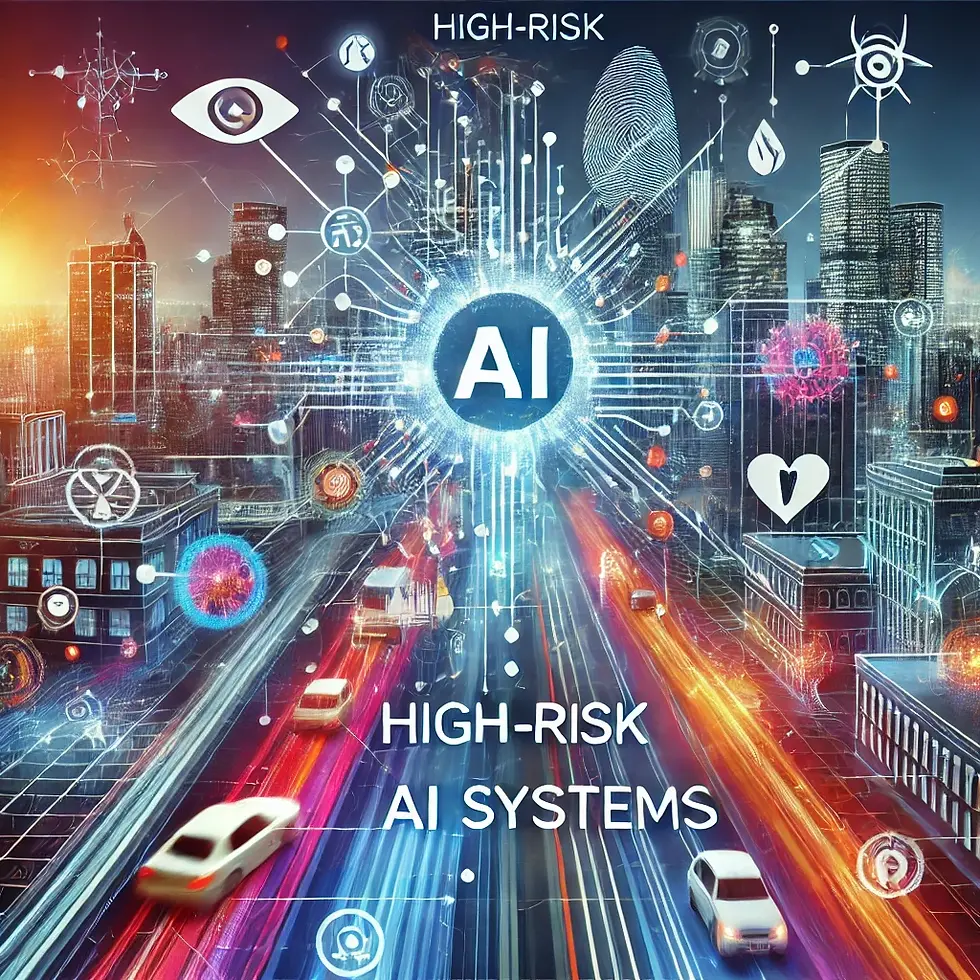
1. Introduction
The European Union's AI Act represents a significant advancement in the regulation of artificial intelligence, setting a framework for the development, deployment, and use of AI systems across the EU. For businesses aiming to operate within this jurisdiction, understanding the implications of this regulation, particularly for high-risk AI systems, is essential.
This deep dive will explore the definition of high-risk AI systems, the applicable parts of the Act, the compliance requirements, and the practical steps businesses can take now and in the future, considering perspectives from both developers and deployers.
2. Defining High-Risk AI Systems
The AI Act categorizes AI systems into several risk levels, with high-risk systems being subject to the most stringent regulations. High-risk AI systems are defined as those used as safety components in products regulated by EU laws listed in Annex I, which require a third-party conformity assessment, or those listed in Annex III, covering use cases such as biometric identification, critical infrastructure management, education, employment, essential services, law enforcement, migration, asylum, border control, and administration of justice. These systems are considered high-risk due to their potential impact on health, safety, and fundamental rights.
3. Examples of High-Risk AI Systems
Understanding high-risk AI systems requires a closer look at specific applications that significantly impact health, safety, and fundamental rights. These systems are subject to stringent regulations to ensure they operate safely, fairly, and transparently.
Below are examples of high-risk AI systems, highlighting the specific challenges and compliance requirements associated with each.
AI in Recruitment
An AI system used for recruitment, which screens and evaluates job applications, falls under the high-risk category. This is because it directly impacts individuals' access to employment opportunities, a fundamental right. Such a system must adhere to strict data governance, transparency, and human oversight requirements to prevent biases and ensure fair treatment of all applicants.
The system must be regularly audited to ensure it is not inadvertently perpetuating discriminatory practices. For example, a recruitment AI that screens resumes must be trained on diverse datasets to avoid bias against any gender, ethnicity, or age group. Additionally, it must provide transparency in how decisions are made, allowing candidates to understand why they were selected or rejected.
AI in Medical Diagnostics
Consider an AI-powered diagnostic tool for early detection of skin cancer. Such a system is classified as high-risk due to its potential impact on patient health and safety. The developers must ensure rigorous testing, maintain detailed technical documentation, and implement robust data governance to ensure the AI system's accuracy and reliability.
For instance, the AI must be trained on a wide range of skin types and conditions to accurately detect cancer across different demographics. It should also include mechanisms for human oversight, allowing healthcare professionals to review and verify AI-generated diagnoses. Continuous monitoring and updates based on real-world performance are essential to maintain its effectiveness and safety.
AI in Credit Scoring
An AI system used for evaluating creditworthiness is another example of a high-risk application. This system must handle sensitive personal data and provide fair and transparent decisions regarding credit access. Developers must implement stringent data governance, risk management, and transparency measures to comply with the AI Act.
For example, an AI used by banks to determine loan eligibility needs to ensure that its decision-making process is free from bias and is based on accurate and relevant financial data. It must also offer clear explanations to users about how their credit scores were calculated and provide avenues for disputing and correcting any errors identified in their credit reports.
AI in Traffic Management
An AI system used for managing traffic flow and safety in a smart city context represents a high-risk application due to its impact on public safety and infrastructure. Deployers must ensure that the system operates reliably, integrates human oversight, and complies with cybersecurity and data governance standards.
For example, an AI system controlling traffic signals must be designed to prevent malfunctions that could lead to accidents. It should be regularly tested under various conditions to ensure its robustness. Human operators should have the ability to override AI decisions in case of emergencies. Additionally, the system should be protected against cyber-attacks that could disrupt city traffic and endanger lives.
AI in Law Enforcement
AI systems used in law enforcement, such as predictive policing tools, also fall into the high-risk category. These systems analyze data to predict potential criminal activity and allocate police resources accordingly. Given their significant impact on civil liberties and the potential for bias, these systems must be developed and deployed with extreme caution.
Developers must ensure that the AI is trained on unbiased data and that its predictions are subject to human review. Transparency is crucial, as communities must be informed about how these systems are used and their potential impacts on privacy and civil rights.
AI in Education
AI systems used in educational settings, such as those for personalized learning or student assessment, are considered high-risk due to their direct impact on students' academic futures. These systems must be designed to support educational equity and avoid reinforcing existing biases.
For instance, an AI system that tailors educational content based on student performance data must ensure that all students have equal opportunities to benefit from its recommendations. Developers should implement safeguards to protect student data privacy and provide educators with tools to understand and intervene in AI-driven decisions when necessary.
AI in Financial Trading
AI systems used in financial trading are high-risk because they can influence market stability and investor outcomes. These systems must be robust, transparent, and subject to strict regulatory oversight.
For example, an AI algorithm that executes high-frequency trades needs to be designed to avoid contributing to market volatility. It should include mechanisms for monitoring and controlling risk in real-time. Developers must ensure that the system operates transparently, providing regulators and stakeholders with clear insights into its decision-making processes.
4. Relevant Provisions of the AI Act
For high-risk AI systems, the AI Act outlines requirements designed to ensure safety, transparency, and accountability. These provisions are detailed in various articles and annexes of the Act and include:
Risk Management System
Article 9 of the AI Act mandates that high-risk AI systems must have a comprehensive risk management system. This involves continuous identification, analysis, estimation, and evaluation of risks associated with the AI system throughout its lifecycle. It also requires implementation of appropriate risk mitigation measures and continuous monitoring and updating of risk management processes.
Data Governance
Article 10 specifies that high-risk AI systems must ensure the quality of datasets used. This includes requirements for data governance practices to guarantee that training, validation, and testing datasets are relevant, representative, free of errors, and complete. Data should be managed in a way that minimizes risks related to bias and discrimination.
Technical Documentation
Article 11 and Annex IV require detailed technical documentation that demonstrates the AI system's compliance with the AI Act. This documentation must cover the design and development process, the system's intended purpose, technical specifications, and a detailed description of how the risk management and data governance requirements are met.
Transparency
Article 13 emphasizes the need for transparency in high-risk AI systems. Providers must ensure that AI systems are designed and developed in a way that allows for the understanding and interpretation of their outputs by users. This includes providing clear and accessible information about the AI system's functionalities, limitations, and how it makes decisions.
Human Oversight
Article 14 outlines the requirements for human oversight in high-risk AI systems. The AI system must be designed to allow for human intervention at appropriate stages to prevent or minimize risks. This can involve real-time monitoring and the ability to override or reverse decisions made by the AI system.
Accuracy and Robustness
Article 15 and Annex VII set out the standards for accuracy, robustness, and cybersecurity of high-risk AI systems. Providers must ensure that their AI systems perform consistently and accurately under all intended conditions of use. Regular testing and validation are required to maintain the system's reliability and performance. Additionally, robust cybersecurity measures must be implemented to protect the system from malicious attacks and data breaches.
Cybersecurity
The cybersecurity requirements, as part of the system's robustness, are also detailed in Article 15. These measures are crucial to ensure the integrity and confidentiality of data processed by high-risk AI systems and to protect against cyber threats.
Quality Management
Article 17 and Annex IX stipulate the need for a quality management system for high-risk AI systems. This includes processes for continuous monitoring, updating, and improving the AI system throughout its lifecycle to ensure ongoing compliance with the AI Act's requirements.
5. Compliance Requirements
5.1 For Developers
Developers bear the primary responsibility for ensuring that high-risk AI systems comply with the AI Act, requiring a multi-faceted approach touching on risk management, data governance, technical documentation, transparency, human oversight, accuracy, robustness, cybersecurity, and quality management.
Implementing a risk management system, as mandated by Article 9, is crucial. This involves establishing a comprehensive framework that includes regular assessments and mitigation strategies. For example, companies developing autonomous vehicle AI should regularly test the system under various conditions, such as different weather and traffic scenarios, to identify potential failure points and implement strategies to mitigate these risks. Monitoring and updating risk management processes based on real-world performance data ensures continuous refinement of risk management strategies.
Data governance requirements, as outlined in Article 10, is essential for maintaining the quality of training data. Developers must use diverse and representative datasets to accurately reflect the populations the AI system will serve. For instance, an AI system for facial recognition should include images from various demographics to avoid biases. Regular audits and updates of data maintain its relevance and accuracy, ensuring decisions remain precise.
Detailed technical documentation, required by Article 11 and Annex IV, involves maintaining comprehensive records of each development stage, from initial design to deployment. For example, an AI used in healthcare should have detailed records of its training, including data types and algorithms. This documentation should also explain the AI system's decision-making processes, such as how an AI used in legal contexts evaluates evidence and reaches conclusions.
Transparency, emphasised in Article 13, requires clear communication about the AI system's functions, capabilities, and limitations. For instance, a customer service chatbot should inform users they are interacting with an AI and explain how their inputs will be used. Developers should create user guides and training materials to help users understand the AI system's operations, ensuring transparency and user education.
Facilitating human oversight, as outlined in Article 14, requires AI systems to be designed to allow human intervention at appropriate stages. For example, an AI system for automated medical diagnosis should enable doctors to review and override AI-generated recommendations. Training staff to oversee and intervene in AI operations is crucial, such as training operators in manufacturing to understand and intervene when the AI malfunctions.
Ensuring accuracy and robustness, mandated by Article 15, requires regular testing and validation of the AI system under different scenarios to ensure consistent performance. An AI system for financial trading should be tested with historical market data to validate its accuracy in predicting trends. The system should adapt to new data, such as updating a transportation AI with new traffic patterns to maintain effectiveness.
Implementing cybersecurity measures, also detailed in Article 15, involves protecting against unauthorised access and conducting regular security audits to identify and address vulnerabilities. For example, an AI system used in critical infrastructure should have multi-layered security measures, including encryption and intrusion detection systems, and undergo frequent security assessments.
Maintaining a quality management system, as required by Article 17 and Annex IX, involves overseeing the AI system from development to deployment and beyond. This ensures continuous compliance with the AI Act and includes processes for continuous monitoring, feedback collection, and updates. For example, an AI in the automotive industry should track the system’s performance and compliance throughout its lifecycle, implementing improvements based on feedback and performance data.
5.2 For Deployers
Deployers, while having fewer obligations than developers, play a crucial role in ensuring the safe and compliant use of high-risk AI systems. Their responsibilities include understanding and managing risks, implementing AI systems according to guidelines, facilitating human oversight, monitoring performance, maintaining cybersecurity measures, and conducting compliance audits.
Understanding and managing risks involves clear communication of potential AI system risks to all relevant stakeholders. For example, in a healthcare setting, ensure all medical staff understand the risks of relying on AI for diagnostics. Training employees to handle and mitigate risks is also essential. For instance, in a financial institution using AI for fraud detection, staff should be trained to respond effectively to AI-generated alerts.
Implementing AI systems according to guidelines requires adherence to the developer’s protocols. For example, when deploying an AI system for automated customer service, ensure the deployment follows the developer’s integration and configuration guidelines. Performing compliance checks ensures the AI system functions as intended, such as verifying that an AI-powered quality control system in manufacturing accurately identifies defects.
To facilitate human oversight establis processes for human intervention and training operators to monitor and intervene in AI operations. For example, in law enforcement using predictive policing, officers should review and override AI-generated predictions. In educational settings using AI for student assessments, teachers should be trained to understand AI-generated results and intervene when necessary to ensure fair evaluations.
Monitoring performance involves continuously tracking the AI system’s real-world outputs and establishing feedback mechanisms to gather user input. For example, in traffic management, continuously monitor the AI system’s decisions to optimise traffic flow without causing disruptions. In retail, gather feedback from store managers on the AI system’s accuracy in predicting stock levels.
Maintaining cybersecurity controls requires regular updates to protect against cyber threats and developing incident response plans. For example, in a critical infrastructure application, regularly update security measures to protect against cyber-attacks. In financial services, have a response plan for data breaches or unauthorised access. It may be beneficial to implement a control framework against a recognised standard for security, such as ISO 27001.
Conducting compliance audits involves regular checks to identify and address compliance gaps. For instance, in healthcare, conduct audits to ensure the AI system used for diagnostics complies with regulatory requirements. Use audit findings to improve the AI system and its deployment processes.
5.3 Practical Steps for Compliance
While it may seem that there is a lot to do, businesses can take several proactive measures to support compliance with the AI Act, particularly for high-risk AI systems. These steps include conducting comprehensive audits, establishing robust documentation protocols, developing AI ethics policies, enhancing data management practices, designing for human oversight, implementing continuous monitoring and improvement processes, and staying informed and engaged.
Conducting a comprehensive audit involves assessing all AI systems to classify them according to the AI Act's risk levels. For example, a company using AI for automated hiring should evaluate the system to determine if it qualifies as high-risk. Developing a detailed roadmap outlining necessary changes and actions for compliance, such as implementing new risk management practices, supports adherence to the Act.
Establishing robust documentation protocols involves creating comprehensive records of AI system development and compliance-related activities. For instance, an AI system used in healthcare diagnostics should have detailed documentation on training datasets and validation steps. Keeping records of risk assessments, mitigation strategies, and audit reports is crucial during regulatory reviews.
Developing an AI ethics policy involves conducting regular ethics reviews at key stages of the AI system's lifecycle and providing staff training on ethical AI practices. For example, a financial institution using AI for credit scoring should review algorithms to ensure they do not discriminate against any group.
Enhancing data management practices involves regular audits of training data to ensure accuracy and bias-free datasets. For instance, an AI system used in law enforcement should be audited to confirm diverse demographic information. Implementing protocols for data updates maintains relevance and accuracy, ensuring precise decisions.
Designing for human oversight involves integrating mechanisms for human intervention and training operators. For example, an AI-powered medical diagnostic tool should enable doctors to review and override AI-generated recommendations. Training staff to monitor and intervene in AI operations is crucial, such as in autonomous driving where human oversight can prevent accidents.
Implementing continuous monitoring and improvement processes involves regular performance monitoring and feedback collection. For instance, in traffic management, continuously track AI system decisions to optimize traffic flow. Create feedback mechanisms to gather user input and real-world performance data for refining the AI system.
Staying informed and engaged involves keeping up-to-date with regulatory developments and best practices. Regularly review updates from EU bodies and industry associations, participate in relevant conferences, and engage with industry peers to share insights and experiences. This helps understand regulatory changes and adapt accordingly.
5.4 Leveraging ISO 42001 for Compliance
Implementing ISO/IEC 42001 can significantly aid businesses in meeting the EU AI Act requirements. This international standard provides a comprehensive framework for AI management systems, emphasising risk management, data governance, and continuous improvement. By adopting ISO/IEC 42001, organisations can create a structured approach to managing their AI systems, ensuring compliance and promoting best practices in AI governance.
6. Conclusion
The EU AI Act's focus on high-risk AI systems underscores the importance of safety, transparency, and accountability. By understanding and adhering to these requirements, businesses can not only ensure compliance but also build trust with their users and stakeholders. Proactive measures, such as adopting ISO/IEC 42001 for AI management, can further support organisations in navigating the complexities of AI regulation and fostering responsible AI innovation.
Comments